Incentive Fraud Is Growing – Here's How To Protect Against It
By Prashant P. Atgur and Arun Menon, Persistent Systems
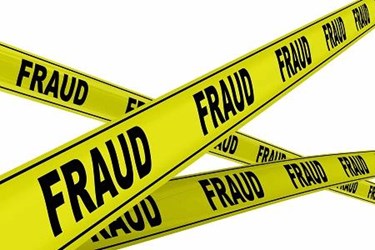
Channel partners are the cornerstone of many companies, generating anywhere between 50-70% of sales. Incentive programs for partners and resellers are the best way to drive performance through partner ecosystems. As billions of dollars flow through structured incentive programs, they become fertile ground for incentive fraud. Organizations that deploy incentive programs must understand how and why incentive fraud takes place and the technology that can help them overcome this problem.
Source: Deloitte Whitepaper - “When Channel Incentives Backfire”
How Extensive Is Incentive Fraud?
In many cases, while basic checks and balances are in place for fraud detection, there is rarely a comprehensive framework built to address sophisticated fraud patterns. It is also becoming increasingly clear that insider fraud is seen in 75% to 80% of the cases. Insiders tend to know the systems and can figure out how to “game it”
The table below — pulled from our conversations with analysts and SMEs in the space — depicts the size of fraudulent transactions as a percentage of incentive payout. As you can see, regions with higher growth potential are more prone to fraud.
Based on the data above, the following table shows the number of fraud dollars in incentive payouts for every $500M payout:
3 Incentive Fraud scenarios
Industry experts have observed what is called the 80/20 rule. Generally speaking, 80% of incentive fraud will take place in just 20% of scenarios. In other words, while there are many ways to commit incentive fraud, most perpetrators exploit the same weaknesses repeatedly.
Below are three of the most common scenarios for incentive fraud:
- Invoice Spamming: Incentive sales data can frequently show startling discrepancies such as out-of-sequence invoice numbers, unusual or abnormal purchases, etc. Invoice spamming can also be achieved by replacing model numbers on invoices with ones of higher payouts. There have also been cases of delaying sales entry/shipping date so that sales become eligible for certain advantageous promotions.
- Fictitious Sales: Because many incentives programs don’t validate customer information, many false claims can go undetected. Fictitious sales can also be in the form of inside sales. In this case, a partner might sell the item to a different store or rep and claim a bonus. On the other side, the store or representative who purchased it will also claim an incentive to essentially double dip.
- Gaming and Internal: In this type of fraud, a user of an incentive system detects a potential bug or flaw in the code and uses it to his or her benefit by either issuing a large number of points or redeeming more points than are available. Another example is keeping the invoice amount below a certain level so that it can pass simple automated systems such as Straight Through Processing. Though these invoices would be for tiny amounts, if generated at a large volume, they can add up.
How Can Technology Be Used To Solve This Problem?
Technology can and will play a very crucial role in building a fraud-resistant incentive program. Organizations need to develop a rules engine which can ensure that eligibility is checked, invoices are tallied, and so on. The new breed of incentive management systems can go a step further by implementing AI to detect anomalies such as a large amount of suspiciously small invoices.
Importantly, these systems track both customer and employee activities which allow companies to detect fraud before things get out of hand. They deploy algorithmic-based analytic tracking methods that monitor transactions that need attention. They also monitor recent behavior, and redemption trends to determine atypical behavior. A supervised machine learning algorithm can categorize and then predict fraudulent attempts. There are also advanced AI systems that can combine supervised and unsupervised learning into a fraud rating or rank.
Designing Better Systems
Currently, most fraud detection systems are based on rules. They help in identifying established patterns of fraud like multiple redemptions generated from a single source IP. However, these systems don’t adapt quickly to new and evolving types of fraud. Detections are not real-time, so the financial loss might have already occurred. Sometimes, the requirements might be complex combinations that are not supported by the rules engine. Moreover, since these rules are added to the business layer, any changes mean building and deploying new updates which will result in downtime.
These drawbacks can be overcome by using a combination of Azure Data factory, event hub, function key vault, Logic Apps’ Machine Learning Studio, SQL database, and so on. They use log data to create and maintain user profiles and use a fraud classification model to produce a predictive score. This uses Azure functions which are serverless, scale out easily, and can be scheduled.
The heart of this system is an AI/ML model that has fraud scenarios fed into it. The model is trained to spot such scenarios. All the incentive claims data is run through the model and processed to flag potential fraudulent transactions for the equivalent of a fraud officer for further investigation. As more and more scenarios are fed and the model gets more robust training, the accuracy of the entire system will improve.
Conclusion
Incentive fraud detection and analytics are important areas for any organization that is leveraging channel partners for sales. With the proper technology, organizations can prevent fraud and save precious dollars. It also has the added benefit of ensuring easily conducted audits and compliance of systems and processes.
About The Authors
Prashant P. Atgur is a Senior Engineering Partner at Persistent Systems, where he heads the delivery unit in the Digital Product Engineering space. In this role, Prashant partners with customers for joint innovation, in areas of Digitization, AI/ML, Blockchain, and relevant technologies. Before joining Persistent, Prashant worked in a regional leadership role at IBM, and over five years as an Enterprise Program Manager at FedEx. Prashant holds an MBA from Dowling College.
Arun Menon is a Delivery Manager and SME in Loyalty, Rewards and Incentive Applications, at Persistent Systems. Arun has 10+ years of experience in consulting, pre-sales, and customer service along with leading a center of excellence around enterprise application implementation, professional services, and network management & systems.